Artificial intelligence (AI) methods increase precision, efficiency and safety in a number of areas, especially in activities that are too dangerous or monotonous to be performed by humans. A look at their possible applications in automotive error diagnosis shows their potential to free mechanics from many time-consuming and error-prone processes in the future.
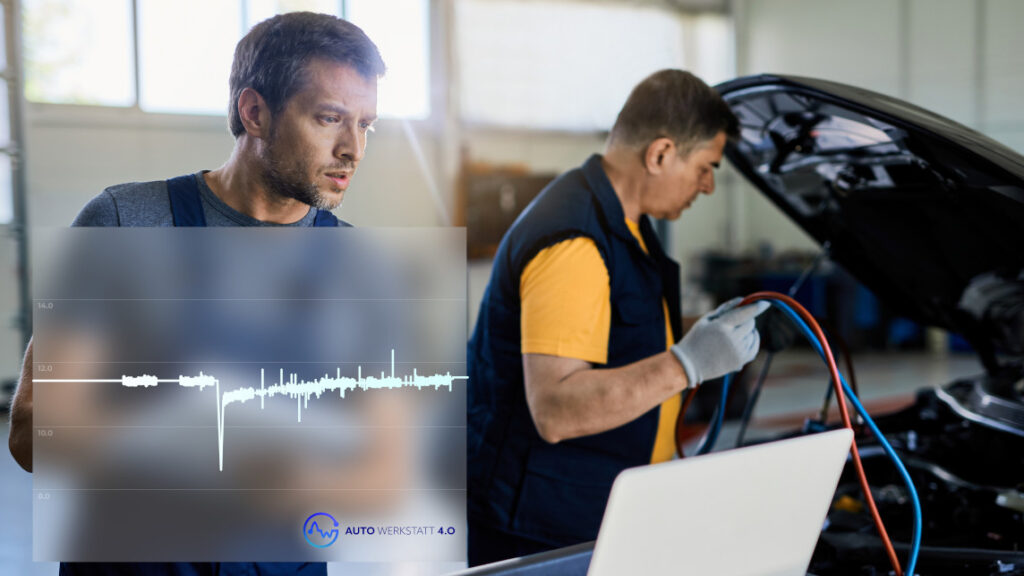
By Tim Bohne, Researcher, German Research Center for Artificial Intelligence (DFKI)
It is nothing new that artificial intelligence methods help to extract meaning from and recognise correlations between data that is complex and unmanageable for humans. The project Car Repair 4.0 uses this to address the increasing complexity and diversity of modern vehicles, which pose a significant challenge for their diagnosis. It researches differentiated, targeted and machine learning-based error diagnosis for motor vehicles and creates a platform for the secure exchange of industry-specific data and AI models as a Gaia-X use case.
AI and Car Repair 4.0
AI offers diverse potential in the automotive sector. There are already a few projects that use AI methods for vehicle diagnostics. In 2019, for example, vehicle manufacturers developed a system that detects anomalies in audio signals recorded in the engine compartment. The developers showed that the accuracy of their system significantly exceeds that of human experts. Another option that the automotive industry is increasingly turning to is predictive maintenance. This involves analysing a large amount of process information and sensor data during operation in order to detect and prevent problematic developments in the future, e.g. through a preventive car check. The keyword is needs-based (data-based) maintenance.
Many parallels can also be drawn with the medical field, where AI methods are also increasingly being used, e.g. in analysing the results of imaging procedures with the aim of identifying patterns in them that are associated with certain diseases. Essentially, it is always about improving the precision of the analysis or diagnosis. Although, in most cases, the decision is made by humans, it helps considerably to start from a good suggestion and check it for plausibility. In order to be able to interpret and classify these suggestions, the focus is increasingly shifting from black-box models to methods of eXplainable Artificial Intelligence (XAI), which make not only the best possible predictions but also ones that are comprehensible to humans. The aim is not to replace human experts but to provide them with a tool that supports them in their diagnostic work. This helps, among other things, to concentrate valuable human working time on relevant activities and compensate for the shortage of skilled workers.
Machine learning-based vehicle diagnostics and anomaly detection
The process of AI-supported vehicle diagnostics in the Car Repair 4.0 project requires the integration of a large number of components. First, the error context is recorded (customer complaints, metadata, vehicle history information, on-board diagnostic log). On the basis of this information, a knowledge graph, which will be created in the course of the project, will be used to record and structure diagnosis-relevant knowledge in order to find out which components in the vehicle need to be analysed in more detail in the present context. An oscilloscope measurement is carried out for these components if they can be reasonably diagnosed with an oscilloscope. Subsequently, the recordings are classified using machine learning methods (deep learning). The resulting oscillograms are finally fed into a classification model that has been trained beforehand on the basis of an extensive data set and which assesses whether the respective recording contains anomalies.
Root cause analysis to determine the actual defect
If an anomaly is identified in this way, the error is isolated by recursively looking at the cause-effect relationships in the vehicle, which are also part of the knowledge graph. After all, in technological systems, one rarely has to deal with errors that occur completely independently of other components in the system. Typically, there are cascading paths where a problem starts at one component and then spreads to other components. The real interest is in the root cause, i.e. the actual cause whose determination is based on the modelled cause-effect relationships. These are initially collected through expert interviews and perspectively supplemented by relationships that are determined through automated analysis of large data sets. Ultimately, this creates an error path that should provide human experts with information about the problem in the vehicle in question.
Resilient results and reduced error-proneness through explainable AI
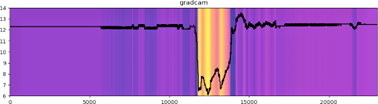
So that car repair shop employees do not have to rely “blindly” on the predictions of the anomaly detection model, XAI methods are used that highlight those areas of the signal that were relevant for the respective decision (see image). In other words: The methods highlight those areas that contributed most to the decision-making of the model. Again: Experts in vehicle diagnostics should be able to recognise quite quickly from such heat maps whether these areas are plausible bases for decision-making or, for example, the result of an over-adjustment. Such procedures not only reduce the susceptibility to errors by enabling people to check the result, but also increase the creditability of the models. Such a “human-in-the-loop” approach, in which the human can contradict implausible recommendations of the system at any time, shows that the system does not replace the human, but merely supports them as a repair assistance system. In summary, the system helps to compensate for the shortage of skilled workers, increases the efficiency and precision of diagnosis and opens up the possibility of preserving expert knowledge for future generations through the resulting knowledge graph.
Errors in the vehicle do not necessarily manifest themselves in a single measurement on a component but may well arise from the specific interaction of various synchronously recorded signals. This is where multi-channel oscilloscopes and the resulting multivariate time series data promise informative analysis possibilities, which will be considered in the course of the project. Furthermore, if a sufficiently large database of past diagnostic procedures is available, statistical conclusions can be drawn as to which procedures and recommendations are most efficient. For example, costly steps and measures could only be recommended if they have a high probability of success in the given context. In this way, unnecessary work steps could be avoided and the effort in the car repair shop could be reduced.
Did you enjoy reading this article? Then subscribe to our newsletter and receive regular updates on related topics and on the Car Repair 4.0 project, and discuss this and similar exciting topics with us on LinkedIn.